Kaikoura earthquake: Collecting social media
Want to know how many times this image was referenced in our Kaikoura Twitter crawl? Read on!
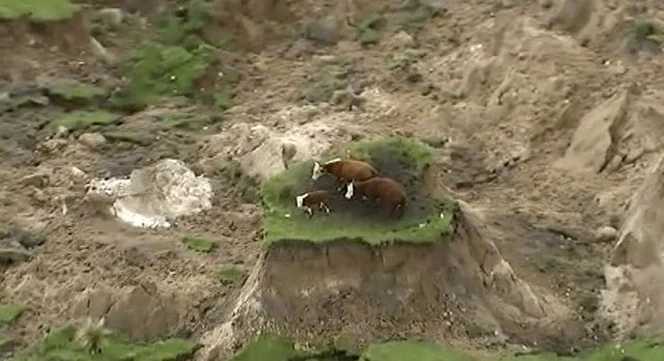
The event
On November 14, 2016 a magnitude 7.8 earthquake struck near the tourist town of Kaikoura. Two people died in the earthquake. Road and rail routes to Kaikoura were cut off and many tourists stranded in Kaikoura had to be evacuated by air or sea. Waiau, Seddon and Ward were also affected. In Wellington, several buildings were damaged, some beyond repair.
The earthquake was documented almost immediately on Twitter. We felt it was important to capture some of this commentary as a record of what occurred during that time.
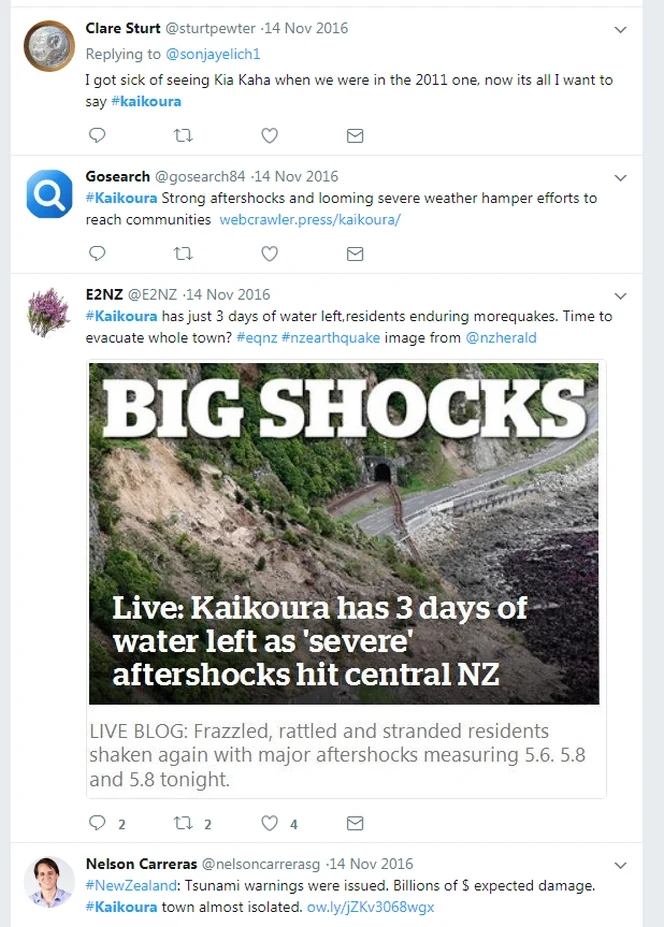
Some of the tweets from the day of the earthquake.
Background
Earlier in 2016 we were looking for an opportunity to run a social media pilot project. We wanted to capture social media commentary on significant events to prevent gaps in our collections. Part of our collecting strategy is to capture websites relating to significant New Zealand events.
At the time we were harvesting websites for the local body elections. We initially considered that an election-related hashtag crawl on Twitter could be useful since Twitter hashtags are often created around events. We chose Twitter because their terms and conditions were favourable towards crawling data using their API. However, we found very few people were using hashtags in the local body elections.
Then the Kaikoura earthquake occurred and we knew this would be an important event to document. And so it became the focus of our pilot Twitter hashtag crawl.
Scope of the crawl
Twitter API’s enable you to capture content from the previous 7 days, so that’s the window of opportunity to capture content from the beginning of a significant event. That 7-day window was helpful, because our work at the Library was disrupted for a few days due to the earthquake. Ben O'Brien, our new Digital Preservation Web Engineer ran the crawl for two weeks to capture the commentary relating to the immediate aftermath of the earthquake.
Search criteria for the crawl
We used the most obvious hashtags and search terms that we were observing on Twitter at the time. These were the search terms used for week 1. In week 2 the date was changed to 2016-11-20.
eqnz OR #NZQuake OR #nzearthquake OR #Kaikoura since: 2016-11-13
nz OR Zealand AND quake OR earthquake OR earthquakes since: 2016-11-13
from:WREMOinfo since:2106-11-13
from:geonet since: 2016-11-13
from:NZcivildefence since:2016-11-13
from:geonet since:2016-11-13
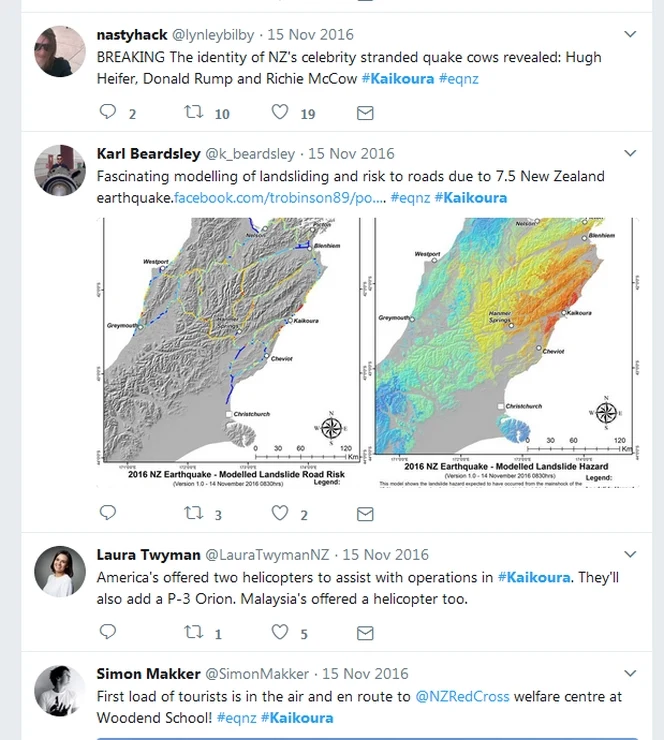
Some tweets that included the hashtag #Kaikoura.
We included three accounts: Geonet (Geological hazard information for New Zealand) which provides aftershock measurements; New Zealand Civil Defence & Emergency Management, the national agency, and WREMO (Wellington Region Emergency Management Office) which provided emergency information that was heavily used during the earthquake.
By the end of two weeks the main activity seemed to have died down so we thought that was a good time to stop the harvest.
Transformation post crawl
Most of us are used to viewing Twitter online using a standard browser. We don’t necessarily think about the underlying data.
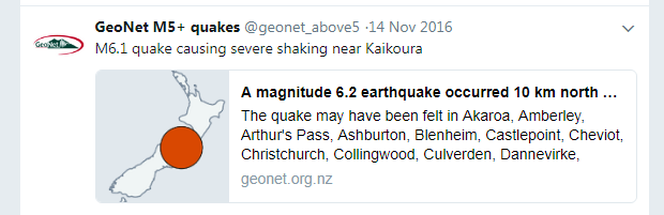
The Twitter crawl uses JSON (JavaScript Object Notation) which is a format for storing and exchanging data .
The raw data is useful for analysing all kinds of information. The example below is some of the metadata from an individual tweet from Geonet:
{"favorited": false, "geo": {"coordinates": [-42.44185582, 173.5807088], "type": "Point"}, "coordinates": {"coordinates": [173.5807088, -42.44185582], "type": "Point"}, "text": "M2.8 quake causing weak shaking near Kaikoura https://t.co/N8VcM02KFS", "possibly\_sensitive": false, "retweet\_count": 1, "lang": "en", "in\_reply\_to\_user\_id\_str": null, "truncated": false, "in\_reply\_to\_screen\_name": null, "retweeted": false, "in\_reply\_to\_status\_id\_str": null, "source": "[GeoNet Quake Push](\"http://www.geonet.org.nz\")", "user": {"following": false, "translator\_type": "none", "profile\_use\_background\_image": true, "time\_zone": "Wellington", "utc\_offset": 46800, "profile\_image\_url": "http://pbs.twimg.com/profile\_images/2225296837/GeoNet\_Project\_logo\_square\_normal.png", "geo\_enabled": true, "followers\_count": 59406, "notifications": false, "profile\_background\_image\_url": "http://pbs.twimg.com/profile\_background\_images/553976237/GeoNet\_Project\_logo\_square.png", "follow\_request\_sent": false, "profile\_background\_image\_url\_https":
Deduplication
Ben deleted any unnecessary files that had already been crawled (deduplication). He combined all JSON from week 1 and deduplicated. He did this again with the JSON from week 2 and then combined all deduplicated JSON from week 1 and 2 and deduplicated again.
Unique Tweets (retweets removed) | Total Tweets (unique or retweeted) | |
---|---|---|
Week 1 | 127,017 | 335,077 |
Week 2 | 16,645 | 41,883 |
Combined | ||
(week 1 and 2 deduplicated) | 137,623 | 356,931 |
Analysis of the crawl
Jay Gattuso, our Digital Preservation Analyst then analysed some of the data for us. We wanted some basic statistics about how many people were tweeting and retweeting, what countries the tweets were coming from and what other file formats were included other than text.
Total Tweets: | 356,931 |
---|---|
Number of unique tweeters: | 16,7964 |
Number of Re-tweets: | 220,508 |
Tweets that have a defined geo location: | 6,988 |
Tweets containing images: | 60,516 (15,781 appear to be unique) |
Tweets containing videos: | 8,312 (224 appear to be unique) |
Location of the Tweets
Country | No. of tweets | % of tweets w/ geo |
---|---|---|
New Zealand | 4663 | 66.7 |
United States | 724 | 10.4 |
United Kingdom | 399 | 5.7 |
Australia | 255 | 3.6 |
Nigeria | 120 | 1.7 |
Canada | 100 | 1.4 |
India | 93 | 1.3 |
Thailand | 71 | 1.0 |
The Netherlands | 44 | 0.6 |
Malaysia | 41 | 0.6 |
As expected the majority of comments came from New Zealand, but a large number came from around the world. Were all these tweets relevant to the Kaikoura earthquake or were people using some of the hashtags for other purposes such as earthquakes occurring elsewhere, or completely off topic? Jay did some further investigation. He discovered that the most retweeted tweet in the harvest was one from ‘realdonaldtrump’. The original tweet wasn’t captured in the harvest, it was the retweet that was captured. It matched on the retweeter’s name “mr__quake” and “Zealand” which was in the text of the original tweet. It was totally unrelated to the Kaikoura earthquake.
That leads us to another question. Should we ‘clean up’ the data before we archive it and only keep tweets relating to the Kaikoura earthquake or should we keep what we captured and let the researcher do the clean-up process when they choose to analyse the data?
The top 15 hashtags
| | ---------- |---------- |
| #eqnz (23,876) | #BREAKING (2,684) |
| #earthquake (16,178) | #kaikoura (2,627) |
| #NewZealand (10,132) | #BREAKING: (2,280) |
| #Kaikoura (4,879) | #earthquakenz (2,025) |
| #Earthquake (4,414) | #EQNZ (1,950) |
| #nzearthquake (3,314) | #quake (1,908) |
| #Tsunami (3,049) | #tsunami (1,713) |
| #NZ (2,687) | |
The eqnz hashtag was by far the top trending hashtag. That indicates it might be advisable for us to collect the EQNZ Twitter account itself as it’s referred to so often. We can’t collect every Twitter account, but we are interested in collecting accounts that are seen as important to society.
Jay tried out some other analytical tools to get an idea of what was in the data.
Word Cloud
Notice the words ‘stranded’ and ‘cow’? That got us thinking about how many times those cows were mentioned!
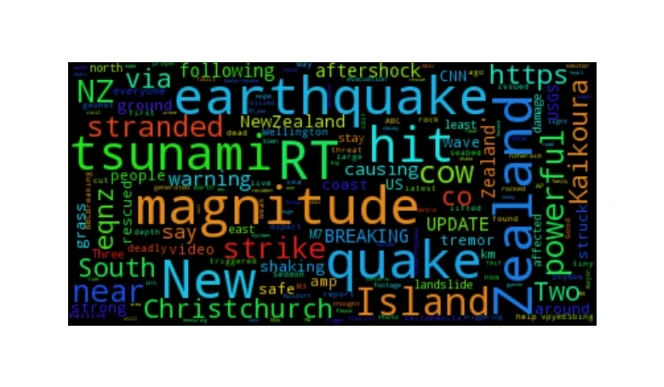
Language
Discovering what languages the Tweets were in proved problematic. Most were in English (131,264; not English 9,323; skipped: 18,449)
Sentiment analysis
We were also interested in sentiment analysis – the Tweeter’s attitude towards the topic. How many tweets were positive, negative, neutral? We found they were pretty evenly divided between positive and negative.
Collecting perspective
Analysing what we have captured is the first stage of the process. Now we need to decide what files we want to preserve in perpetuity, how we archive them and what kind of access to provide. That’s not just from a preservation or archival perspective, but it’s also important that what we collect and the way we collect it is useful for researchers. So if you’re a researcher, we’d be interested in your feedback.
If you’d like to know more about building a social media archive, and the kind of questions that need addressing there are some useful guidelines on Social Feed Manager.
Oh, and how many times were those cows referenced in our Twitter crawl? 28,570 times!
Co-written by Gillian Lee (Coordinator, Web Archives) and Jay Gattuso (Digital Preservation Analyst)
Hi there,
How can we retrieve the dataset??